Meet our new faculty:
Alejandro Schuler
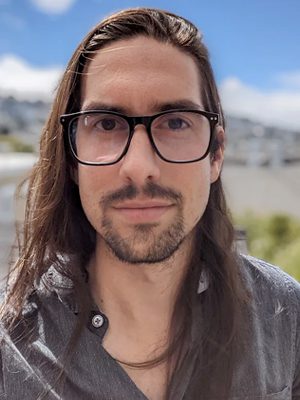
- Discipline: Biostatistics
- Research interests: Nonparametric statistics, causal inference, electronic health records, gradient boosting, power calculation
- Hometown: Cupertino, CA
- Current city: San Francisco
- Pronouns: He/him
- Hobbies: Gravel cycling, guitar, chess, video/board games
In July 2022, Berkeley Public Health welcomed new Assistant Professor in Residence of Biostatistics Alejandro Schuler. Dr. Schuler received his PhD in biomedical informatics from Stanford in 2018, his MS in mechanical engineering from UCLA in 2013, and his BS in mechanical engineering from UCLA in 2012. We asked him about his work and hopes for the semester.
Where did you live and work before you started at UC Berkeley School of Public Health?
I’ve been in San Francisco since 2013. After I finished my PhD, I worked as a data scientist at Kaiser’s Division of Research in Oakland and then I joined an early-stage startup called Unlearn.AI. I came back to academia last year as a postdoc with Mark van der Laan. (Check out his research group’s site here.)
What are you currently working on?
I have a handful of methods development projects in the works.
One of the areas that I’m working in at the moment is power calculation for randomized trials that use covariate adjustment. Adjusting for baseline covariates lets you explain away more variance in the outcome, meaning that you typically get smaller confidence intervals and p-values when you adjust. But it’s usually not clear how to take advantage of that principle to design smaller trials: Instead of having a trial with the same number of people that gets a more accurate answer, you could use fewer people to come to an answer that has the same accuracy. I put a paper out last year that lets you do this if you’re adjusting with a fancy estimator like targeted maximum likelihood.
Now, I’m working on the equivalent for the more commonly-used generalized linear models. The idea is to figure out exactly what minimal set of assumptions and population parameter estimates you need to tell you how many fewer subjects you could use by doing covariate adjustment.
What are your hopes going into your first semester at UC Berkeley School of Public Health?
One thing I’d like to do as soon as possible is build collaborations with faculty in other divisions at BPH. My most creative methods work in the past has come from working on tricky applied problems that came up in the real world. I also love helping domain scientists to actually understand how and why “complex” methods actually work so they can be more creative and accurate in translating their research questions to a mathematical formalism for data analysis. So collaborations are key and most welcome!